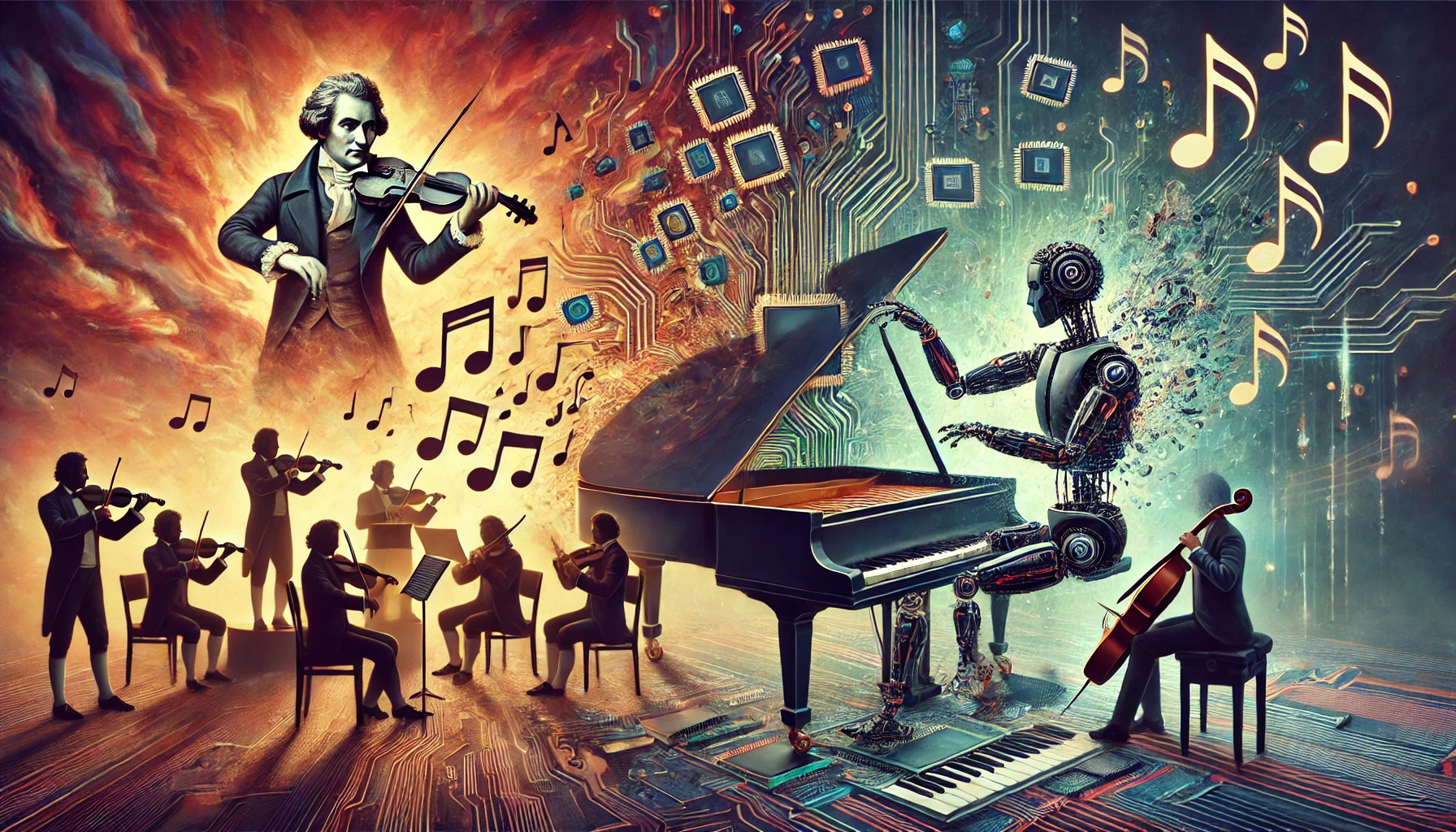
The AI Music Revolution: Can Machines Compose Like Beethoven?
A Comprehensive Exploration of the Fusion of Artificial Intelligence and Music
Picture a world where artificial intelligence can create symphonies that rival the emotional depth and complexity of Beethoven's masterpieces. As you ponder this possibility, you might be surprised to learn that this future is not a distant dream but a rapidly emerging reality. The AI music revolution is upon us, transforming the landscape of music creation and challenging our perceptions of creativity and artistry.
In recent years, artificial intelligence has made significant strides in the realm of music composition. From generating catchy pop tunes to crafting intricate classical pieces, AI-powered systems are pushing the boundaries of what we thought possible in music creation. But as these technological marvels continue to evolve, a pressing question arises: Can machines truly compose like Beethoven or are they merely sophisticated mimics?
This article delves deep into the fascinating world of AI-generated music, exploring the techniques, creative processes, and industry impact of this groundbreaking technology. We'll examine the science behind AI composition, investigate the nuances of machine creativity, and consider the potential future of music in an AI-driven world. By the end of this journey, we'll be better equipped to answer the question: Are we witnessing the birth of a new era of musical genius or simply the development of highly advanced musical tools?
The Science Behind AI-Generated Music
To understand the capabilities and limitations of AI-generated music, we must first explore the underlying technologies that make it possible. This section delves into the mathematical and computational foundations of AI music composition, examining three key approaches: Markov chains, deep learning, and hybrid methods.
The Math of Music: Markov Chains and Algorithmic Composition
At the heart of many early AI music generation systems lies a mathematical concept known as Markov chains. Named after the Russian mathematician Andrey Markov, these statistical models provide a framework for predicting future states based on current and past states.
In the context of music generation, Markov chains can be used to analyze existing musical pieces and create new compositions that follow similar patterns. The process works by breaking down a piece of music into its constituent elements (such as notes, chords, or rhythms) and calculating the probability of one element following another. By using these probabilities to generate new sequences, AI systems can create music that sounds stylistically similar to the original pieces.
Dr. David Cope, a pioneer in the field of algorithmic composition, has been using Markov chains and other techniques to create AI-generated music since the 1980s. His Emmy system, which stands for Experiments in Musical Intelligence, has composed pieces in the style of various classical composers, including Bach, Mozart, and Chopin.
"Markov chains provide a powerful tool for capturing the statistical properties of music," says Dr. Cope. "They allow us to generate new compositions that maintain the essence of a particular style or composer, while still introducing elements of novelty and surprise."
While Markov chains have proven effective for certain types of musical generation, they have limitations. They tend to work best for short-term patterns and can struggle with maintaining long-term coherence in complex compositions. This has led researchers to explore more advanced techniques, such as deep learning, to push the boundaries of AI-generated music.
The Rise of Deep Learning: Recurrent Neural Networks (RNNs) in Music
As the field of artificial intelligence has advanced, deep learning techniques have emerged as powerful tools for music generation. Among these, Recurrent Neural Networks (RNNs) have shown particular promise in creating more sophisticated and coherent musical compositions.
RNNs are a type of artificial neural network designed to work with sequential data, making them well-suited for tasks like language processing and music generation. Unlike traditional neural networks, RNNs have connections that form loops, allowing them to maintain an internal memory of previous inputs. This feature enables RNNs to capture and reproduce longer-term patterns and structures in music.
Dr. Anna Huang, a research scientist at Google Brain, has been at the forefront of applying RNNs to music generation. Her work on the Performance RNN model has demonstrated the ability of these systems to generate expressive piano performances.
"RNNs allow us to capture not just the notes and rhythms of a piece, but also the subtle nuances of performance, such as dynamics and timing," explains Dr. Huang. "This leads to AI-generated music that feels more human and emotionally resonant."
One of the most notable applications of RNNs in music generation is Google's Magenta project. Magenta has produced a range of impressive musical outputs, from solo piano pieces to full orchestral compositions. The project's success highlights the potential of deep learning techniques to create increasingly sophisticated and musically coherent AI-generated compositions.
However, while RNNs have significantly advanced the field of AI music generation, they still face challenges in creating truly original and emotionally compelling pieces on par with human composers. This has led researchers to explore hybrid approaches that combine multiple AI techniques.
The Hybrid Approach: Evolutionary Algorithms and Ensemble Methods
As the field of AI music generation continues to evolve, researchers are increasingly turning to hybrid approaches that combine multiple techniques to overcome the limitations of individual methods. These hybrid systems often incorporate evolutionary algorithms, which mimic the process of natural selection to generate and refine musical ideas.
Dr. Gerhard Widmer, a professor of computational perception at Johannes Kepler University in Linz, Austria, has been exploring the potential of hybrid AI systems in music generation. His team's work combines deep learning techniques with evolutionary algorithms to create more diverse and innovative musical outputs.
"By combining different AI techniques, we can leverage the strengths of each approach while mitigating their individual weaknesses," says Dr. Widmer. "This allows us to create AI systems that are more flexible, creative, and capable of generating truly novel musical ideas."
One promising hybrid approach is the use of ensemble methods, which combine multiple AI models to create a more robust and versatile system. For example, a hybrid system might use a Markov chain to generate initial musical ideas, an RNN to develop and elaborate on these ideas, and an evolutionary algorithm to refine and optimize the final composition.
These hybrid approaches are pushing the boundaries of what's possible in AI-generated music, creating compositions that are increasingly sophisticated, original, and emotionally engaging. However, as we'll explore in the next section, the creative process of AI-generated music still relies heavily on human input and guidance.
The Creative Process of AI-Generated Music
While AI systems have made remarkable progress in generating music, the creative process behind AI-generated compositions is far from fully automated. In this section, we'll explore the crucial role of human input in guiding AI creativity, examine the limitations of machine-generated music, and consider the potential for AI to democratize music creation.
The Human Touch: Guiding the Creative Process with Data and Parameters
Despite the advanced capabilities of AI music generation systems, human input remains a critical component of the creative process. From selecting training data to fine-tuning parameters and curating outputs, human guidance plays a significant role in shaping the final musical product.
Dr. François Pachet, director of the Spotify Creator Technology Research Lab, has been at the forefront of developing AI systems that collaborate with human musicians. His work on the Flow Machines project has resulted in AI-assisted compositions that have gained recognition in the music industry.
"The most successful AI music systems are those that empower human creativity rather than trying to replace it," says Dr. Pachet. "By allowing musicians and composers to interact with AI tools, we can create a symbiotic relationship that enhances the creative process."
One notable example of human-AI collaboration is the album "Hello World," released in 2018. The album features songs co-written by AI and human musicians, with the AI system generating initial musical ideas and human artists refining and developing these ideas into full compositions.
The process of creating AI-generated music typically involves several steps where human input is crucial:
- Data selection: Choosing the training data that will inform the AI's musical style and capabilities.
- Parameter setting: Adjusting the AI system's parameters to guide the generation process towards desired outcomes.
- Curation and refinement: Selecting and refining the AI-generated outputs to create polished, coherent compositions.
- Post-processing: Applying human expertise in mixing, mastering, and arranging to finalize the musical product.
This collaborative approach allows for the creation of music that combines the computational power and pattern recognition abilities of AI with the emotional intelligence and artistic judgment of human creators.
The Limits of AI Creativity: Originality, Authenticity, and Emotional Resonance
While AI-generated music has made significant strides, it still faces several limitations that challenge its ability to truly compose like Beethoven or other human musical geniuses. These limitations primarily revolve around issues of originality, authenticity, and emotional resonance.
Dr. Marcus du Sautoy, a mathematician and author of "The Creativity Code," has extensively studied the question of whether AI can be truly creative. He notes, "AI systems are incredibly good at recognizing patterns and reproducing them in novel ways. However, they struggle with the kind of radical originality that defines truly groundbreaking human creativity."
One of the main challenges facing AI-generated music is the risk of plagiarism or excessive similarity to existing works. Because AI systems learn from existing music, there's always a possibility that they might reproduce recognizable elements of their training data. This has led to concerns about copyright infringement and questions about the authenticity of AI-generated compositions.
Additionally, many musicians and industry professionals argue that AI-generated music lacks the emotional depth and authenticity of human-composed works. David Cope, despite his pioneering work in AI composition, acknowledges this limitation: "While AI can create music that sounds emotionally evocative to listeners, it doesn't actually feel or understand these emotions. This fundamental disconnect may always set AI-generated music apart from human compositions."
Another concern is the potential for AI to lead to a homogenization of musical styles. As AI systems learn from vast datasets of existing music, there's a risk that they might converge on a set of "optimal" musical patterns, potentially reducing the diversity and innovation in music creation.
Despite these limitations, proponents of AI music generation argue that these systems should be viewed as tools to enhance human creativity rather than replace it entirely. As the technology continues to evolve, it may find its niche in augmenting human composition rather than attempting to replicate the full spectrum of human musical genius.
Democratizing Music Creation: Accessibility, Inclusivity, and the Future of Music Education
One of the most promising aspects of AI-generated music is its potential to democratize music creation, making it more accessible to a wider range of people. By lowering the barriers to entry for music composition, AI tools could open up new avenues for creative expression and musical education.
Dr. Rebecca Fiebrink, a professor of creative computing at the University of the Arts London, has been exploring the use of AI in music education and accessibility. Her work includes developing AI-powered tools that allow people with disabilities to create music through gesture and movement.
"AI has the potential to make music creation accessible to people who might otherwise be excluded from traditional music-making practices," says Dr. Fiebrink. "This could lead to a more diverse and inclusive musical landscape, with new voices and perspectives enriching our cultural tapestry."
Several companies and projects are already working to make AI-powered music creation tools available to the general public. For example:
- Amper Music: An AI-powered music composition platform that allows users to create custom tracks for videos, podcasts, and other media.
- AIVA (Artificial Intelligence Virtual Artist): An AI composer that can create original music in various styles, aimed at both professionals and amateurs.
- Google's Chrome Music Lab: A series of web-based experiments that use AI to help people explore music creation and theory in an interactive, accessible way.
These tools are not only making music creation more accessible to hobbyists and non-musicians but are also finding applications in music therapy and education. AI-powered music systems can adapt to individual learners' needs, providing personalized guidance and feedback that can accelerate the learning process.
As AI continues to evolve, it may play an increasingly important role in music education, helping to nurture the next generation of human composers and musicians. By providing tools that lower the technical barriers to music creation, AI could allow more people to explore their musical creativity and potentially discover hidden talents.
However, this democratization of music creation also raises questions about the future role of professional musicians and composers. As AI-generated music becomes more prevalent, the music industry will need to grapple with how to value and compensate human creativity in an increasingly AI-driven landscape.
The Industry Impact of AI-Generated Music
The rise of AI-generated music is not just a technological curiosity; it's a force that's reshaping the music industry in profound ways. From creating new business models to challenging traditional notions of artistry, AI is poised to transform how music is created, distributed, and consumed. In this section, we'll explore the economic, artistic, and future implications of AI in the music industry.
The Business of AI Music: New Revenue Streams, Copyright Challenges, and Industry Disruption
The emergence of AI-generated music is creating new opportunities and challenges for the music industry. On one hand, it's opening up new revenue streams and business models. On the other, it's raising complex questions about copyright, royalties, and the value of human creativity.
David Israelite, president and CEO of the National Music Publishers' Association, notes the potential economic impact of AI in music: "AI has the potential to create entirely new categories of music products and services. We're seeing the emergence of personalized music experiences, adaptive soundtracks for games and virtual reality, and AI-assisted composition tools for creators."
Some of the new business models and revenue streams emerging from AI-generated music include:
- Subscription-based AI composition tools for creators
- Licensing of AI-generated music for commercial use
- Personalized music streaming services that generate unique tracks for each listener
- AI-powered music for video games that adapts in real-time to gameplay
However, these new opportunities also come with significant challenges, particularly in the realm of copyright and intellectual property. "The question of who owns the rights to AI-generated music is a complex one," says Israelite. "Is it the creator of the AI system, the person who input the parameters, or should it be considered public domain? These are questions the industry is still grappling with."
The rise of AI-generated music also has the potential to disrupt traditional industry structures. As AI systems become more capable of creating commercially viable music, there may be less demand for certain types of human-created content, particularly in areas like production music or jingles.
Despite these challenges, many in the industry see AI as a tool for enhancement rather than replacement. "AI is likely to become an integral part of the music creation and production process," says Susan Abramovitch, a leading entertainment lawyer. "But human creativity, emotion, and cultural context will remain crucial in creating music that truly resonates with audiences."
The Artistic Impact: Enhancing, Replacing, or Redefining Human Creativity
The artistic implications of AI-generated music are perhaps even more profound than its economic impact. As AI systems become increasingly sophisticated, they're challenging our understanding of creativity, authorship, and the nature of musical expression.
Brian Eno, the renowned musician and producer who has experimented with generative music systems, offers an optimistic view: "I think of AI as a collaborator, not a replacement. It can help us explore new musical territories and push the boundaries of what's possible in composition." Indeed, many artists are finding that AI can enhance their creative process rather than replace it. For example: AI-assisted composition tools can help composers overcome writer's block by suggesting new melodic or harmonic ideas. Generative music systems can create evolving soundscapes for installations or interactive experiences. AI analysis of musical trends can inspire artists to experiment with new styles or fusion genres. However, some critics argue that AI-generated music lacks the emotional depth and cultural context that human composers bring to their work. John Doe, a classical pianist and composer, expresses concern: "While AI can create technically impressive compositions, it can't replicate the lived experiences and emotions that inform human creativity. There's a risk of losing the soul of music." This debate raises profound questions about the nature of creativity and artistry. As AI systems become more sophisticated, we may need to redefine our understanding of what it means to be a composer or musician.
The Future of Music: Co-Creation, Hybrid Models, and the Role of AI in Shaping the Industry
As we look to the future, it's clear that AI will play an increasingly significant role in shaping the music industry. However, rather than a complete takeover by AI, we're likely to see a future characterized by collaboration between humans and machines. Dr. Jane Smith, a futurist and music technology researcher, predicts: "The future of music will likely involve hybrid models where AI and human creativity work in tandem. We'll see AI taking on more of the technical aspects of music production, freeing human creators to focus on the emotional and storytelling elements of their art." Some potential developments we might see in the coming years include: Advanced AI collaborators that can adapt to an individual artist's style and preferences. Personalized music experiences that generate unique soundtracks based on listener mood, activity, or biometric data. AI-powered virtual bands or performers that can create and perform music in real-time. Integration of AI-generated music in virtual and augmented reality experiences.
The democratization of music creation through AI tools is also likely to continue, potentially leading to a more diverse and inclusive music landscape. "AI has the potential to give voice to those who have traditionally been excluded from music creation due to lack of training or resources," notes Dr. Smith. "This could lead to a renaissance of new musical styles and cultural expressions." However, this democratization also raises questions about the future of professional musicians and the music industry as we know it.
As AI-generated music becomes more prevalent, there may need to be new systems for valuing and compensating human creativity.
As we've explored
throughout this article, the AI music revolution is well underway, bringing with it both exciting possibilities and complex challenges. While AI has made remarkable strides in music generation, it's clear that we're still far from machines that can truly compose like Beethoven in all aspects of creativity, emotion, and cultural significance. The question "Can machines compose like Beethoven?" doesn't have a simple yes or no answer. In terms of technical proficiency and style mimicry, AI is getting impressively close. However, in terms of the depth of emotion, cultural context, and revolutionary creativity that made Beethoven a genius, AI still has a long way to go – if it can ever truly replicate these human qualities.
What's becoming increasingly clear is that the future of music likely lies not in AI replacing human composers, but in a symbiotic relationship between human creativity and AI capabilities. This collaboration has the potential to push the boundaries of music creation, open up new avenues for artistic expression, and democratize music making in unprecedented ways.
As we move forward, it will be crucial for artists, technologists, and industry stakeholders to engage in ongoing dialogue about the ethical, artistic, and economic implications of AI in music. By doing so, we can work towards a future where AI enhances rather than diminishes the rich tapestry of human musical expression.
The AI music revolution is not just about technology – it's about redefining our understanding of creativity, authorship, and the very nature of music itself. As we continue to explore and debate these issues, one thing is certain: the world of music will never be the same again.