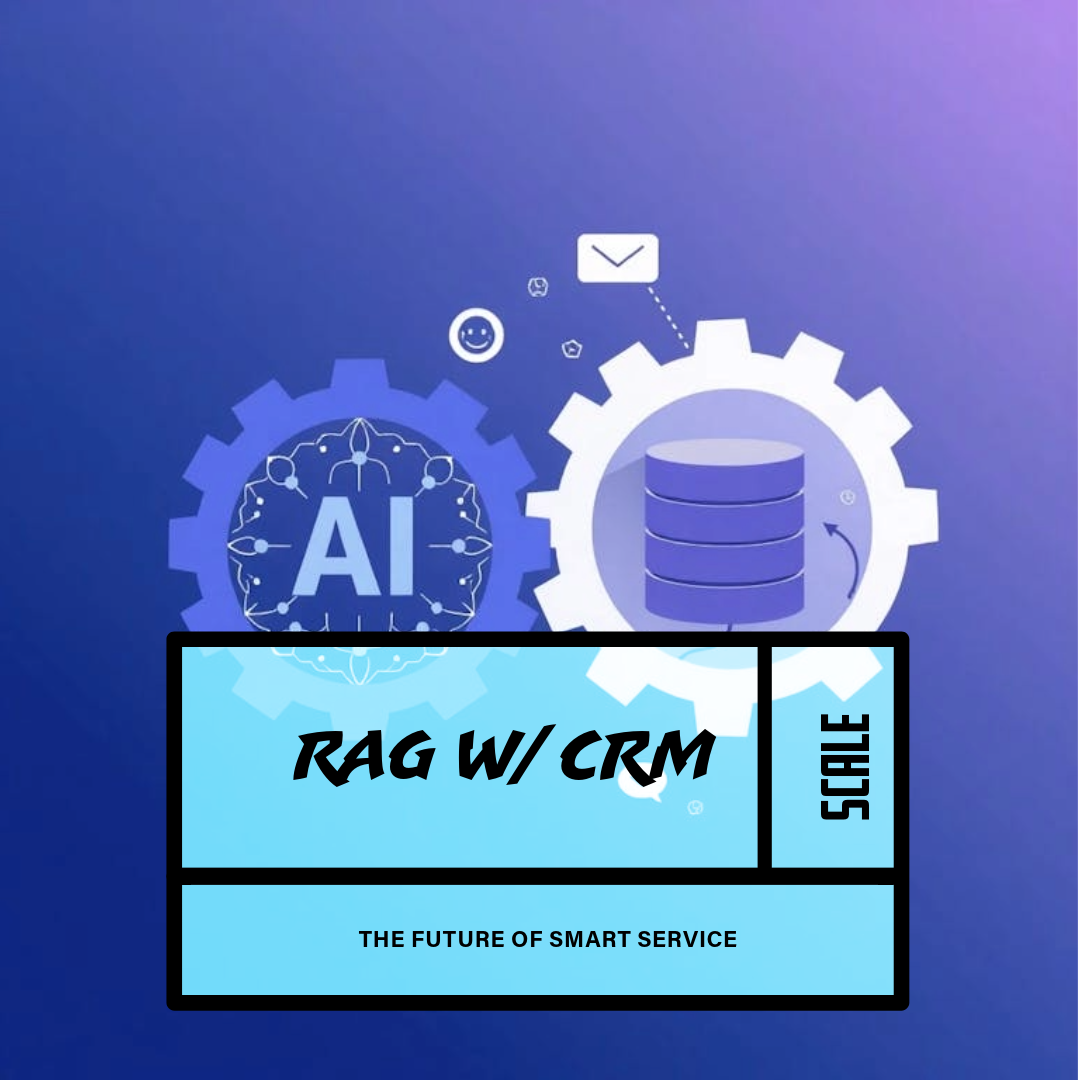
Next Level of CRM with Retrieval-Augmented Generation (RAG)
Ever wondered why, despite all the advancements in AI, our interactions with language models can still feel…lacking? It’s like we’ve got this supercomputer, but we’re only using it to make memes and count letters. Today, I want to dive deep into Retrieval-augmented generation (RAG) and how it’s set to revolutionize Customer Relationship Management (CRM) across industries.
The Nuts and Bolts of RAG
First off, let’s get technical—but not too technical, I promise. Traditional language models predict the next word in a sequence based solely on their training data. This is where they hit a wall: they’re limited to the information they’ve been fed up until their last update. Enter RAG.
RAG combines the generative prowess of language models with a retrieval system that accesses a vast external knowledge base in real-time. Think of it as giving your AI assistant the ability to Google things on the fly, but in a way that’s seamlessly integrated into its responses.
Here’s how it works:
- Query Understanding: The model takes your input and grasps the intent.
- Information Retrieval: It searches an external database or document store to find the most relevant pieces of information.
- Response Generation: It uses both the retrieved information and its inherent language understanding to generate a response.
This means your AI isn’t just smart—it’s informed.
Why Prompting Alone Isn’t Cutting It
Mastering Prompting and Prompt Engineering Guide have been our go-to methods for coaxing better responses from language models. We’ve been crafting elaborate prompts, setting up context, and even using chain-of-thought techniques to get more accurate answers.
Here’s the kicker: no matter how cleverly you engineer a prompt, a model can’t provide information it doesn’t have. It’s like asking a librarian about a book that hasn’t been published yet.
RAG sidesteps this limitation by fetching up-to-date information right when it’s needed.
RAG Meets CRM: A Perfect Union
In the realm of CRM, timely and accurate information is king. Customers expect immediate answers that are tailored to their specific needs. Here’s where RAG shines:
- Personalized Interactions: By accessing real-time data, RAG can provide responses that are highly personalized, improving customer satisfaction.
- Consistency Across Channels: Whether it’s chatbots, email responses, or voice assistants, RAG ensures that all customer touchpoints have the same level of up-to-date information.
- Scalability: With RAG, you can scale customer interactions without scaling your team proportionally. The AI handles the heavy lifting.
Technical Advantages of RAG in CRM
- Dynamic Knowledge Integration: RAG allows for the incorporation of dynamic data sources like current inventory levels, recent customer interactions, and the latest product updates.
- Reduced Hallucinations: One of the challenges with language models is their tendency to “hallucinate” or generate plausible but incorrect information. By grounding responses in retrieved data, RAG minimizes this issue.
- Compliance and Accuracy: In industries like finance or healthcare, providing accurate information isn’t just preferable—it’s mandatory. RAG ensures compliance by referencing approved data sources during response generation.
Combining RAG with Advanced Prompt Techniques
While RAG is a game-changer, combining it with sophisticated prompt engineering takes things to the next level. By structuring prompts that guide the retrieval process, we can fine-tune the balance between the model’s generative capabilities and the specificity of the retrieved information.
For example:
- Contextual Prompts: Crafting prompts that include context cues can help the model retrieve more relevant information.
- Instruction Tuning: Adjusting prompts to instruct the model on how to use the retrieved data can lead to more coherent and useful responses.
Challenges and Considerations
Implementing RAG isn’t without its hurdles:
- Infrastructure Requirements: RAG systems need access to large-scale, searchable databases, which can be resource-intensive.
- Latency Issues: Real-time retrieval can introduce delays. Optimizing the retrieval process is crucial for maintaining quick response times.
- Data Security: Accessing and handling customer data requires robust security measures to protect sensitive information.
Here’s the good news: advancements in technology are rapidly addressing these challenges. With cloud computing and optimized algorithms, the barriers to entry are lowering.
Industries Poised for Transformation
Every sector that relies on customer interaction stands to benefit:
- E-commerce: Providing real-time product recommendations and support.
- Healthcare: Offering patients immediate, accurate information while ensuring compliance.
- Finance: Delivering up-to-date financial advice and account information securely.
Wrapping It Up
When you’re talking about customer engagement, sticking to outdated methods is like using a flip phone in the smartphone era. Retrieval-Augmented Generation isn’t just an upgrade; it’s a paradigm shift.
By integrating RAG with your CRM, you’re not just keeping up with the times—you’re setting the pace. It’s about providing value, enhancing customer experiences, and staying ahead of the competition.
Ready to dive deeper? Let’s connect and explore how we can tailor RAG solutions to elevate your CRM strategy.